The research entitled "A combined ionic Lewis acid descriptor and machine-learning approach to prediction of efficient oxygen reduction electrodes for ceramic fuel cells" was recently published in Nature Energy (IF: 67.439) by Xie Heping, an academician from the college of Civil and Transportation Engineering, Shenzhen University, with his PhD student Zhai Shuo as corresponding and first author respectively, and Professor Ni Meng from Hong Kong Polytechnic University and Professor Shao Zongping from Nanjing University of Technology as co-corresponding authors. The research combines machine learning, theoretical computation and ceramic solid oxide development to develop an experimentally validated machine learning screening technique for cathode materials, achieving a major breakthrough in screening highly active cathode materials for solid oxide fuel cells.
The clean and efficient use of coal and the promotion of the coal revolution are of great strategic importance to the reform of Chinese energy structure! At present, modern coal-fired power plants are limited by the Carnot cycle, and the coal consumption per unit of power generation remains high. At the same time, it is difficult to break the technical bottleneck of large CO2 emissions inherent to coal power generation, and it is impossible to truly realize the clean and low-carbon utilization of coal. Xie Heping's team has proposed and is working on the "Near Zero Carbon Emission Direct Coal Fuel Cell (DCFC) Power Generation Technology" (CN114284533A). This technology can break the limits of the Carnot cycle and convert the chemical energy of modified coal directly into electricity through the electrochemical oxidation process instead of combustion, while achieving secondary energy utilization of CO2 in-situ in the system. The DCFC is based on a solid oxide fuel cell, where the cathode provides the oxygen ions required for the carbon oxidation reaction and the intrinsic activity of the material plays a decisive role in the kinetic reaction rate of the oxygen reduction reaction. However, conventional materials design, characterisation and testing rely on an inefficient trial and error process that often requires long research cycles.
Based on the above research thinking, this research innovatively combines machine learning, theoretical calculations and solid oxide fuel cell cathode material design to achieve rapid and effective screening of highly active cathode materials from a large calcium titanite fraction. The study elucidates the mechanism of the Lewis acidity modulation strategy of chalcogenide oxides to enhance intrinsic activity, and reveals the mechanism by which the polarization distribution of Lewis acidity in the A- and B-site ions causes the deflection of electron pairs, which in turn reduces the generation energy and migration energy barriers of oxygen vacancies. The results provide the theoretical basis and technical support for the team's ongoing research on "near-zero carbon emission direct coal fuel cell power generation technology".
Direct link to the paper:
https://www.nature.com/articles/s41560-022-01098-3
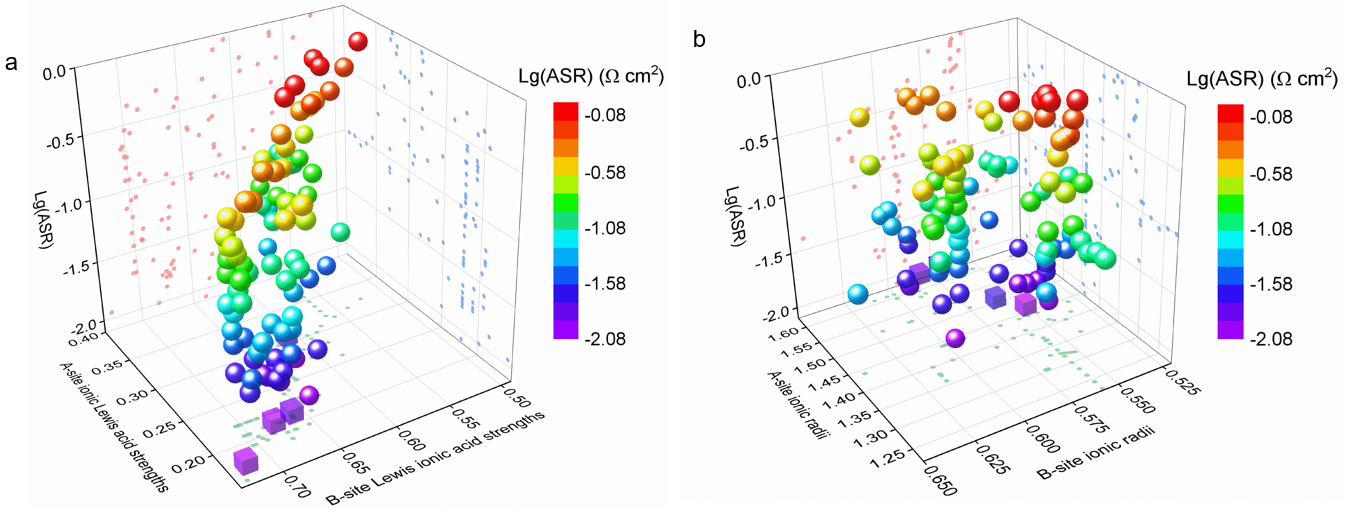
Fig.1 Overall workflow diagram for the development of solid oxide fuel cell cathode
materials based on machine learning
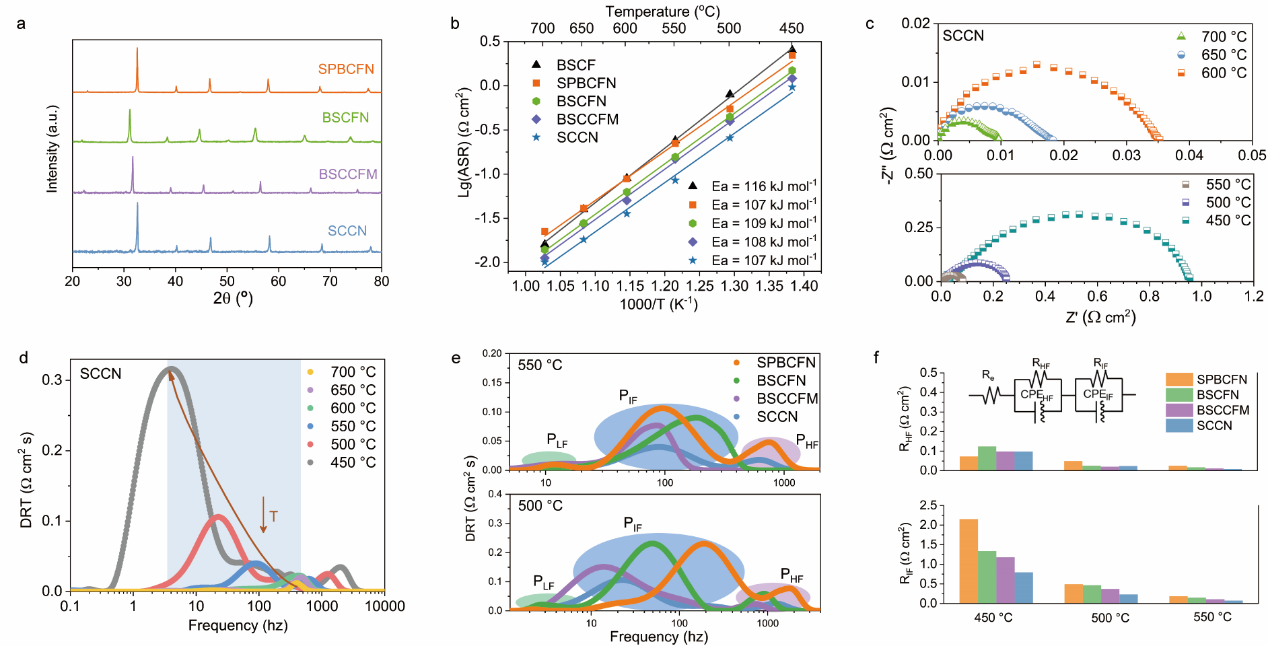
Fig.2 Structure and electrochemical properties of highly reactive chalcogenide cathode
materials
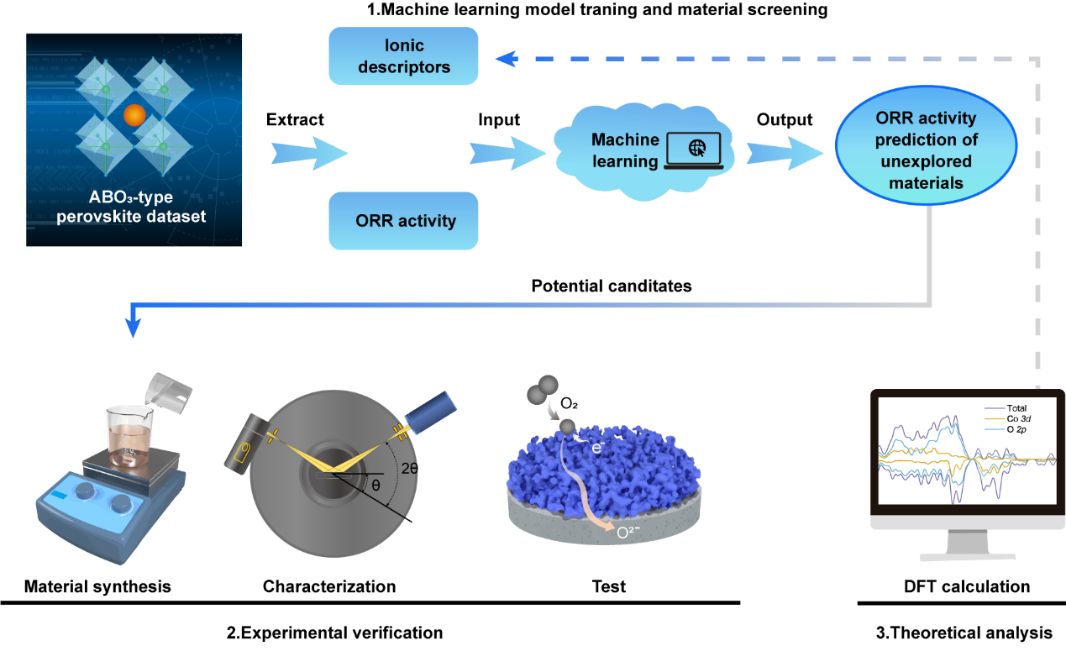
Fig. 3 Three-dimensional visualization of the correlation between the intrinsic activity of chacogenide
cathode materials and the strength of Lewis acidity as a function of ionic radius