报告题目:
Landslide Susceptibility Mapping using Physics-Guided Machine Learning (PGML): An Application to A Debris Flow Event in Colorado Front Range
报告人:
Tong Qiu, Ph.D., P.E., F.ASCE, Professor
Department of Civil and Environmental Engineering
The Pennsylvania State University
报告时间:2024年5月14日(星期二)15:30-18:30
报告地点:深圳大学沧海校区致理楼L1座10楼1007会议室
报告人简介:
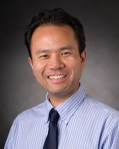
Dr. Qiu received his B.S. degree in hydraulic engineering from Tsinghua University, China, in 2000 and M.S. and Ph.D. degrees in civil engineering with a focus in geotechnical engineering from UCLA in 2002 and 2005, respectively. Before joining the faculty at The Pennsylvania State University in 2010, he was a project engineer in southern California and a faculty member at Clarkson University. Dr. Qiu was an associate editor for the Journal of Geotechnical and Geoenvironmental Engineering, ASCE, and the International Journal of Geomechanics, ASCE, from 2014 to 2023. He was an elected board member of the United States Universities Council on Geotechnical Education and Research. Dr. Qiu received the Excellent Paper Award from the International Journal of Geomechanics, ASCE, and the Faculty Early Career Development Award (CAREER) from the National Science Foundation and is an elected Fellow of ASCE.
报告简介:
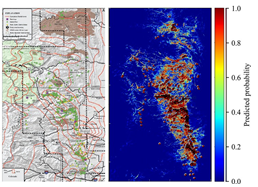
Physics-guided machine learning (PGML) is a new paradigm for applying machine learning (ML) techniques to solve engineering problems. This talk will demonstrate the capability of PGML to improve traditional ML models for mapping/predicting debris flow hazards through a case study of the debris flows triggered by a storm event in the Colorado Front Range. The spatial distribution of the debris flows was assessed using three distinct classes of methods: a physics-based model, a traditional ML model, and a PGML model. For the physics-based model, the infinite-slope model with submerged conditions was used to calculate the factor of safety and assess the debris flow risk. For the PGML model, a physics-guided loss function was implemented to integrate debris flow physics into traditional ML models. It was found that PGML can improve ML model performance for mapping the debris flow hazards by improving physics consistency and making ML models more generalizable from one ecoregion to another.